☰
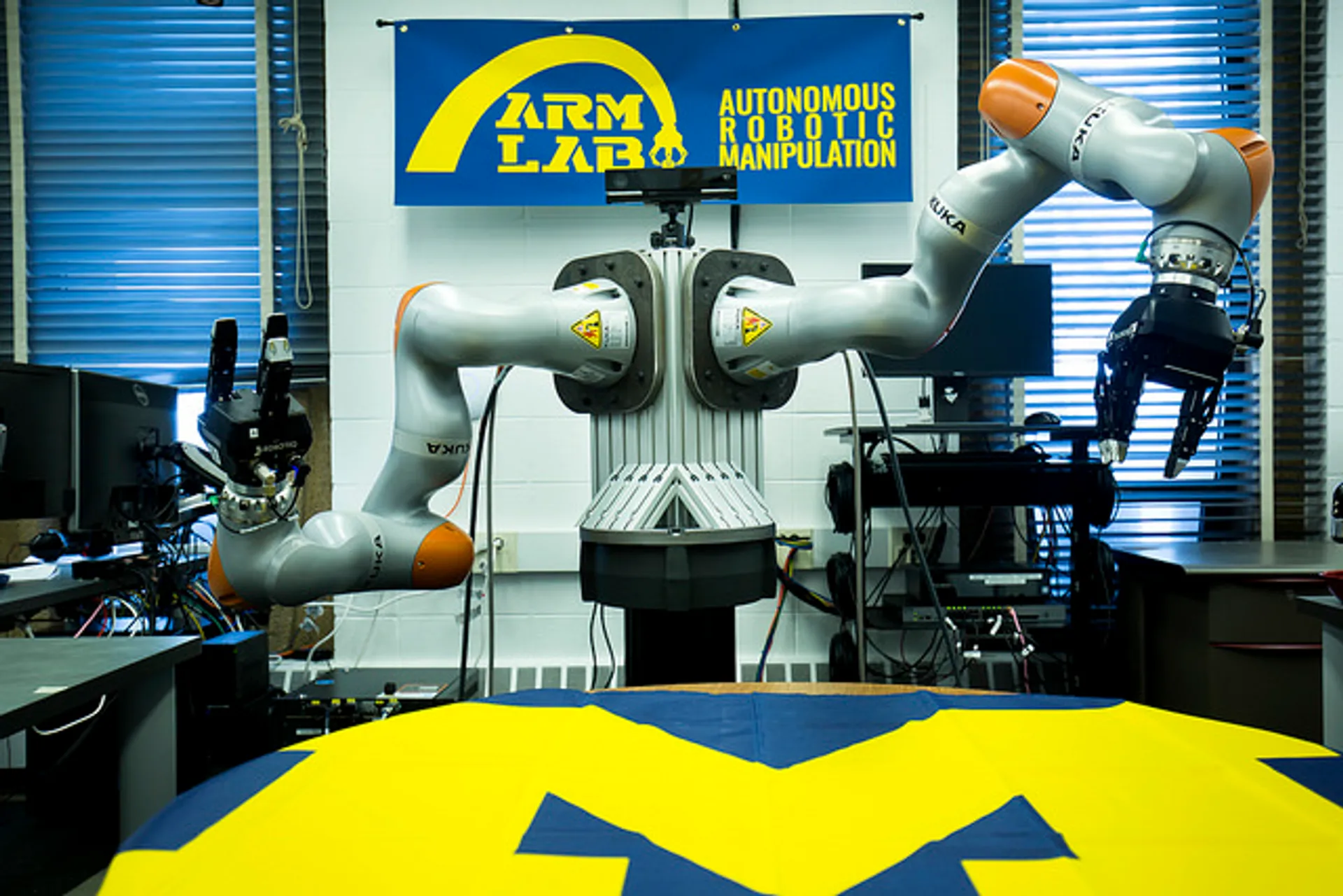
News about Perception & Manipulation
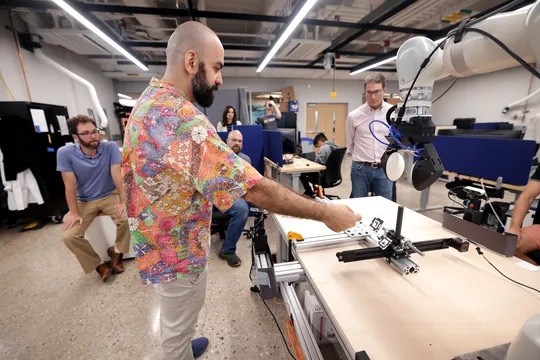
Nima Fazeli awarded NSF CAREER grant
June 7, 2024
Nima Fazeli, assistant professor of robotics, was awarded the National Science Foundation’s Faculty Early Career Development (CAREER) grant for a project “to realize intelligent and dexterous robots that seamlessly integrate vision and touch.
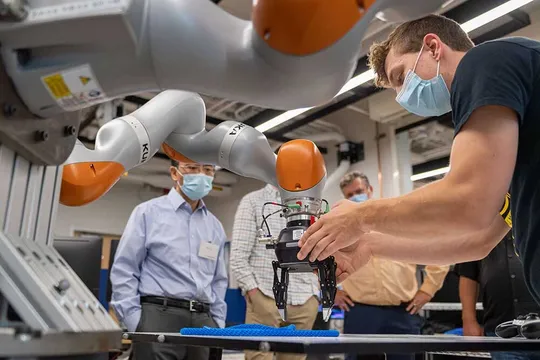
'Fake' data helps robots learn the ropes faster
June 29, 2022
In a step toward robots that can learn on the fly like humans do, a new approach expands training data sets for robots that work with soft objects like ropes and fabrics, or in cluttered environments.
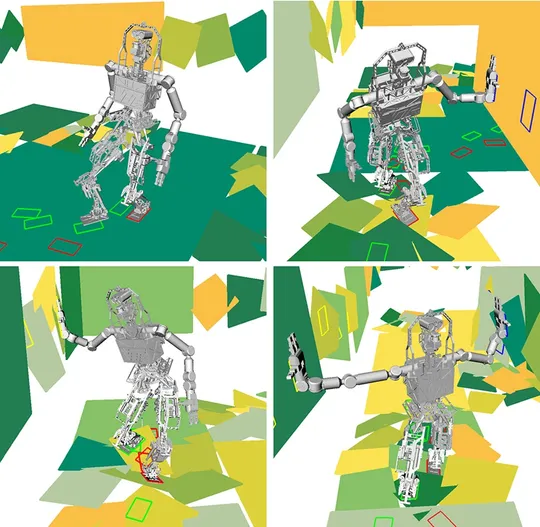
Rubble-roving robots use hands and feet to navigate treacherous terrain
August 13, 2021
A new way for robots to predict when they can’t trust their models, and to recover when they find that their model is unreliable.
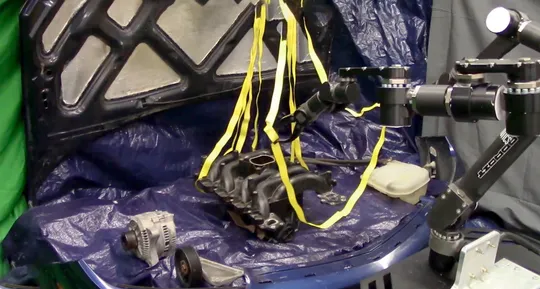
Helping robots learn what they can and can’t do in new situations
May 19, 2021
To overcome this problem, University of Michigan researchers have created a way for robots to predict when they can’t trust their models, and to recover when they find that their model is unreliable.
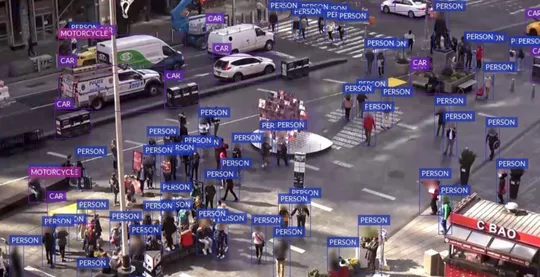
Using computer vision to track social distancing
April 15, 2020
A University of Michigan startup is tracking social distancing behaviors in real time at some of the most visited places in the world.
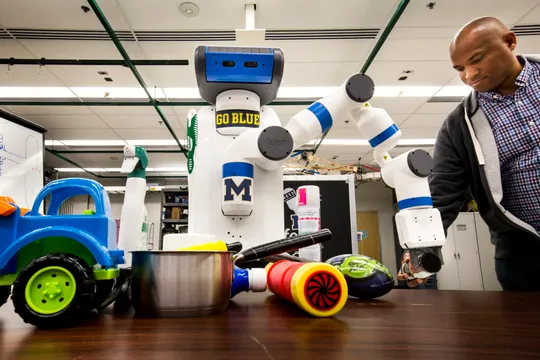
A quicker eye for robotics to help in our cluttered, human environments
May 23, 2019
A University of Michigan team has developed an algorithm that lets machines perceive their environments orders of magnitude faster than similar previous approaches.